The competitive analysis of PPC (Pay Per Click) is that he sees what your competitors do with paid ads on platforms such as Google Ads, so you can do it better. It helps you discover which keywords spend money on what their ads and target websites look like, and how much budget they can work.
If you can’t afford to spend a week on manual analysis or you doubt that you have sufficient experience to do it well, the next best option is a enormous language model (LLM), such as chatgpt, Claude or Gemini. All you need to know is where to look for PPC data and a good hint – that’s what I will assist you.
Here are some examples from a ready project that I have already created using this method.
The first example is an executive summary, ensuring a brief review of the analysis.
And here CHATGPT performs analyzes of the overlap of keywords and analyzes of the keyword gap. You can also download full CSV results.
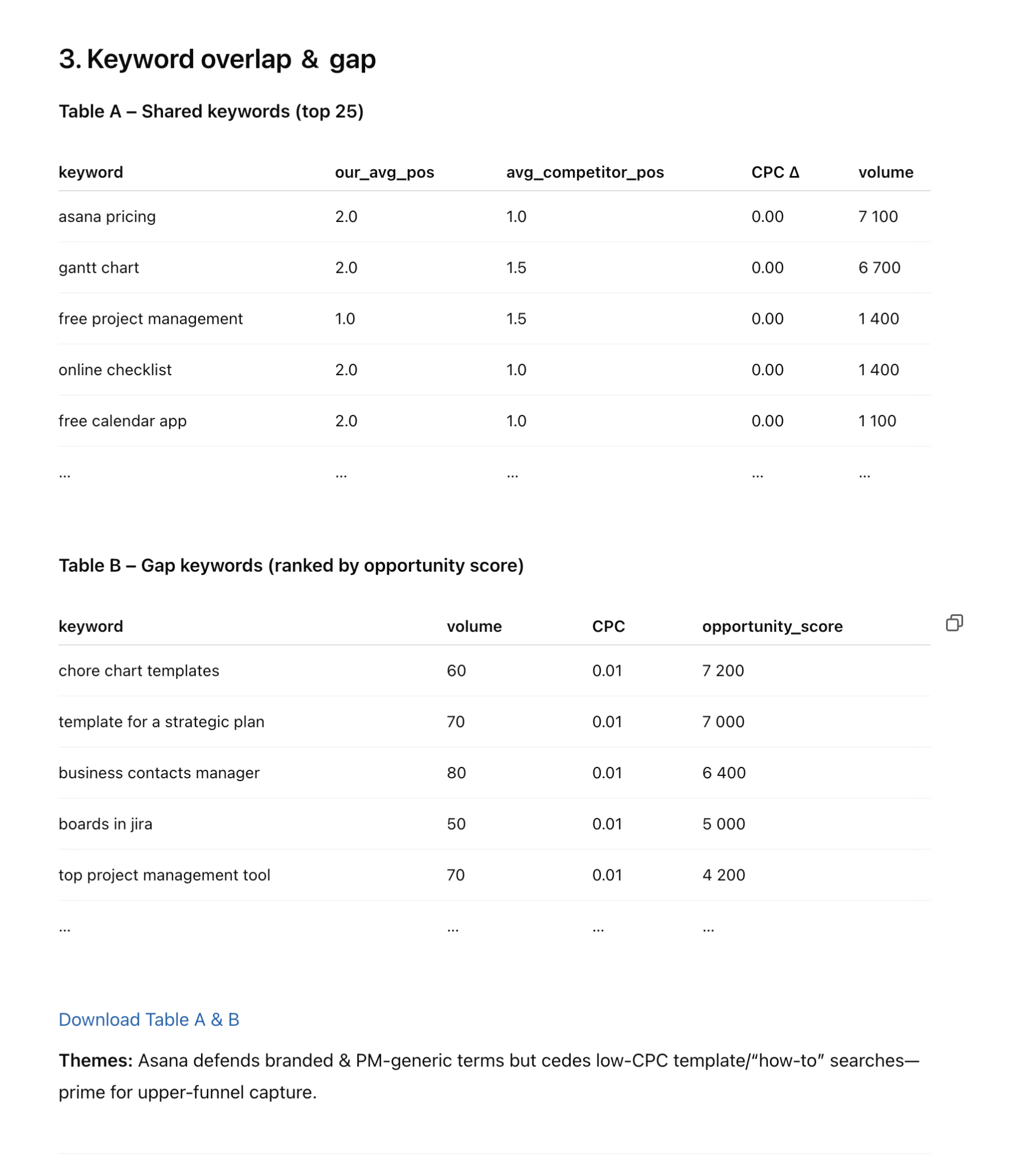
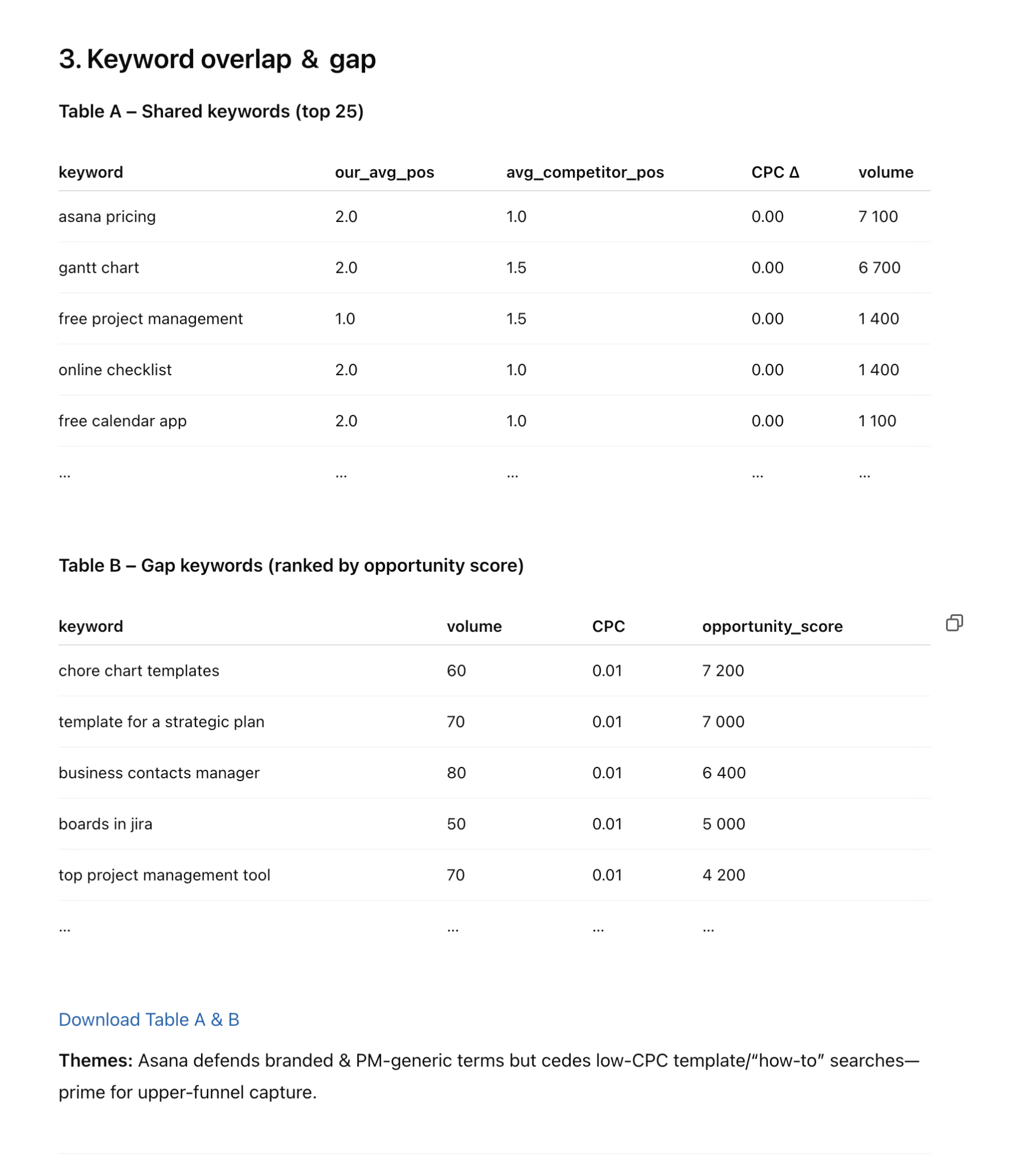
My PPC analysis plan connects Ahrefs and ChatgPT to provide a full picture of advertising competitors in Google ads and assist quickly improve your own.
Thanks to Ahrefs you will see exactly which keywords are bidding on your competitors, the countries they aim, and what ads and the target pages they exploit – something that you can’t do with the Google keyword planner.
You will also receive estimated daily, weekly and monthly advertising expenses to assess their aggressiveness and point patterns.
Then ChatgPT enters to identify keyword gaps (keywords they bid on, but not), pull out key insights from the data and suggest uncomplicated victories that you can act quickly. After the analysis, you can even ask you to build a full PPC action plan, including recommendations regarding the target sites and a better copy of ads to boost performance.
Let’s start!
At this stage, we will decide what to consider in a competitive analysis, collect data on paid competitors’ movement and find significant trends in their expenses.
First, make a list of websites of your competitors. To make sure you don’t lose them, you can check the organic competition report on the site’s audit. There are chances that the sites that want to survive will also bid on the same keywords in Google ads.
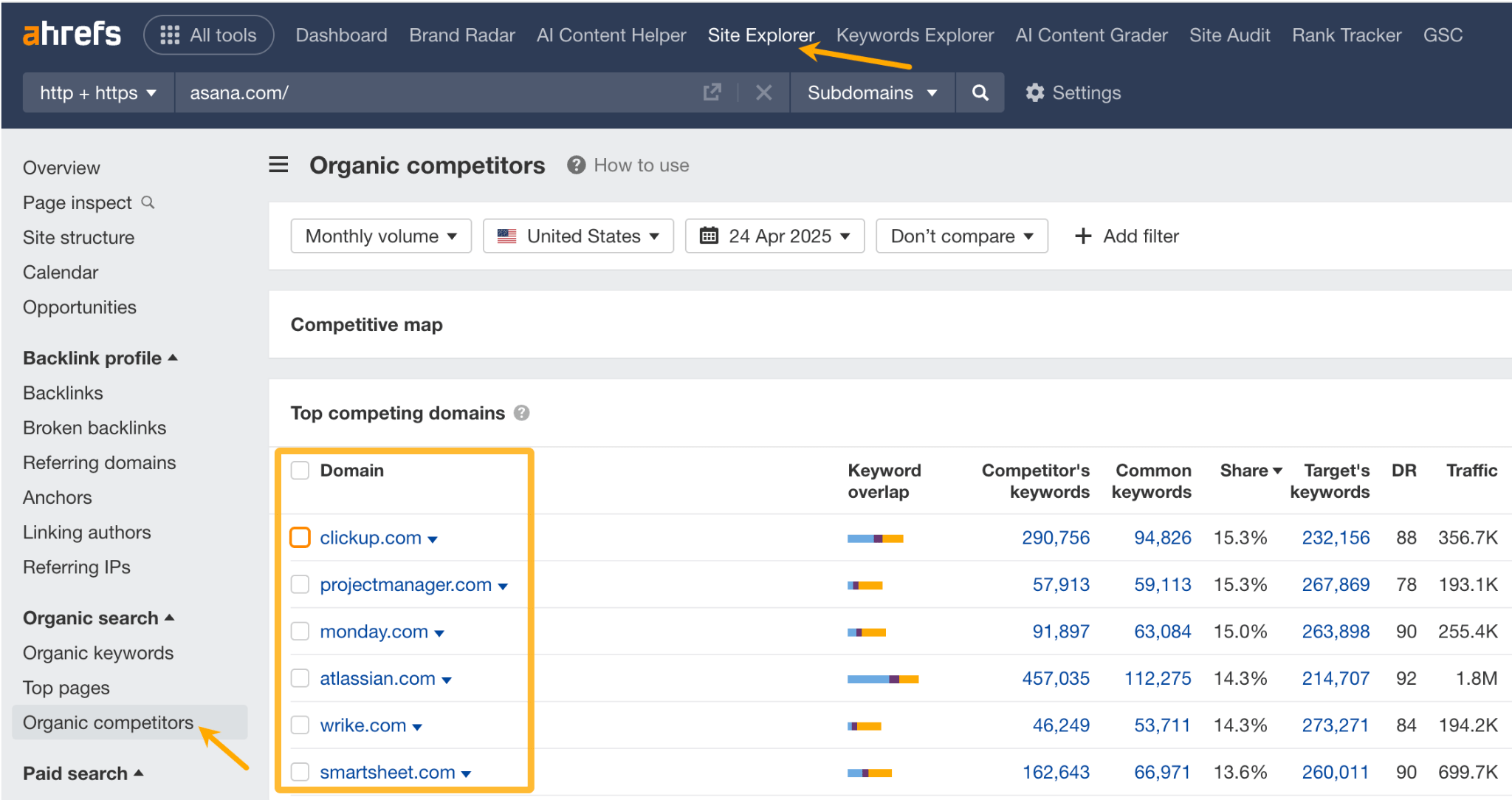
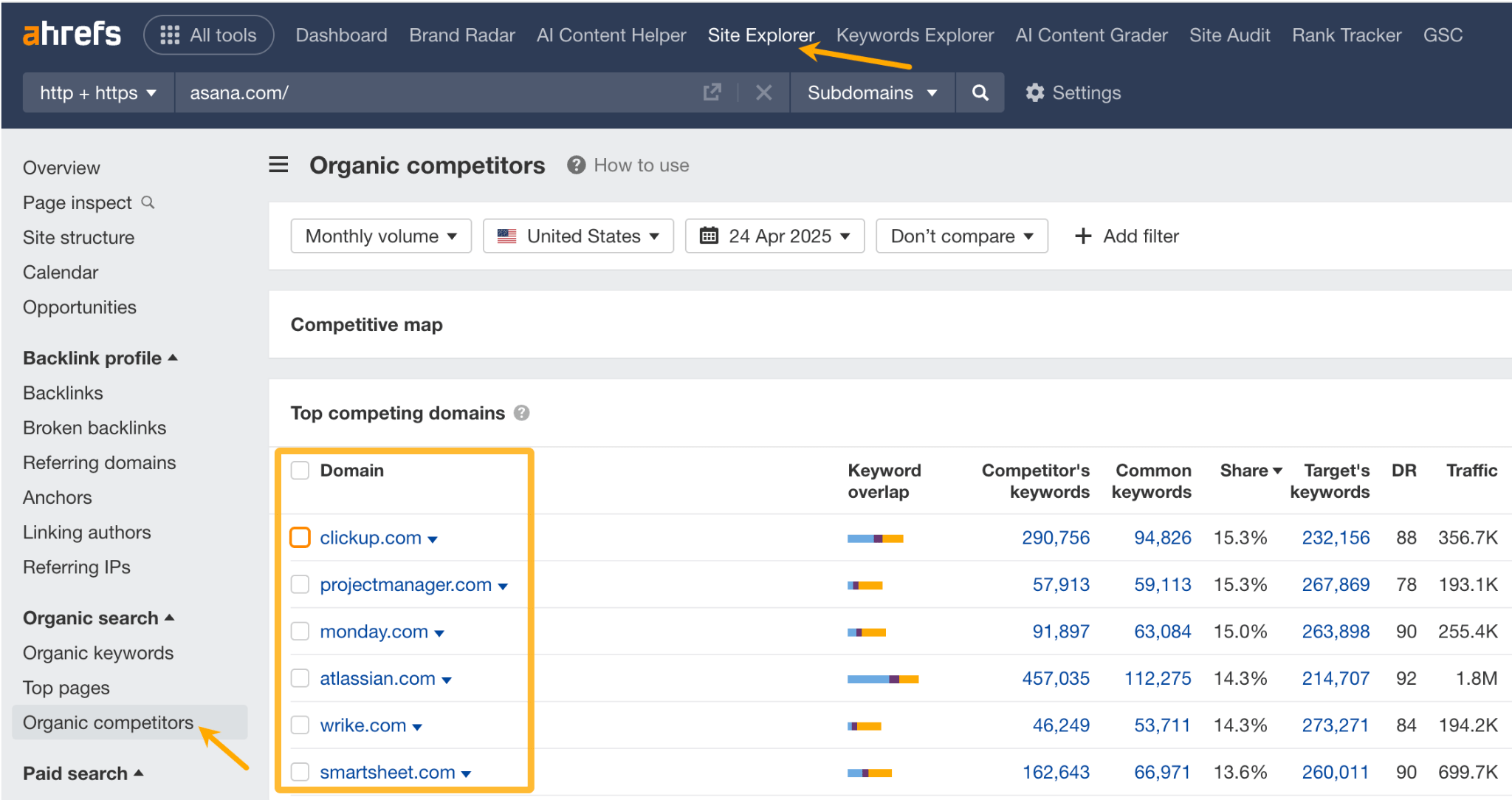
Now connect the competition domains with your party analysis. Export the results.
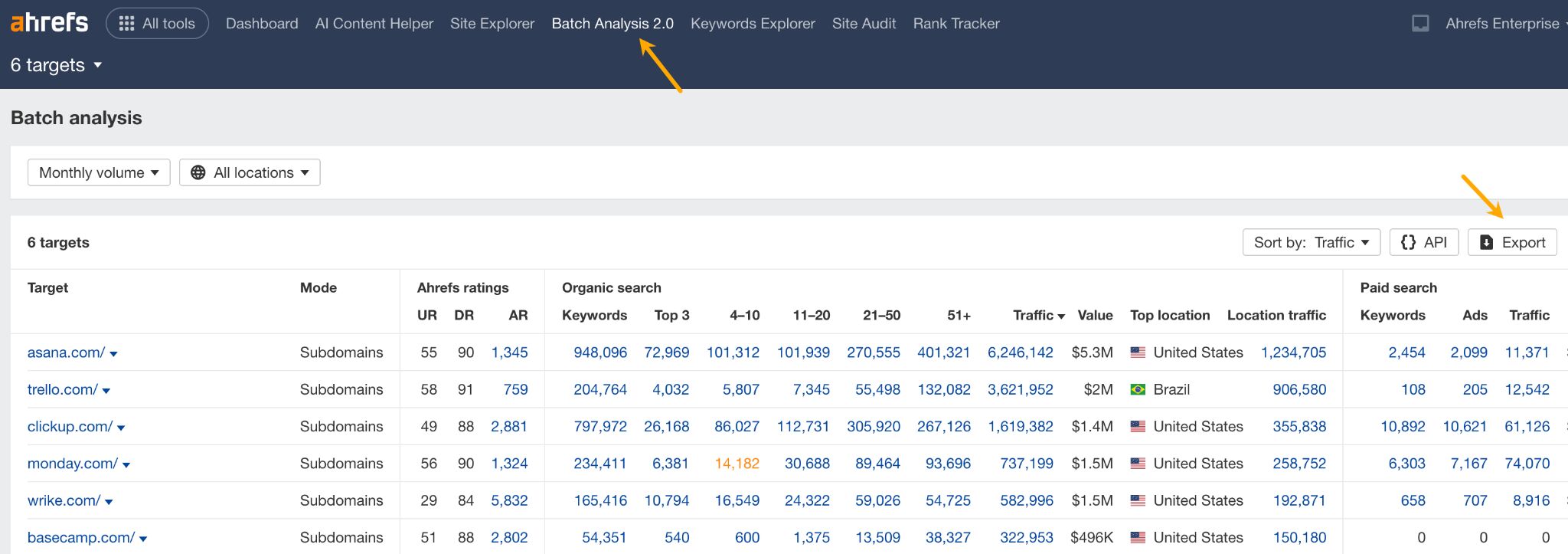
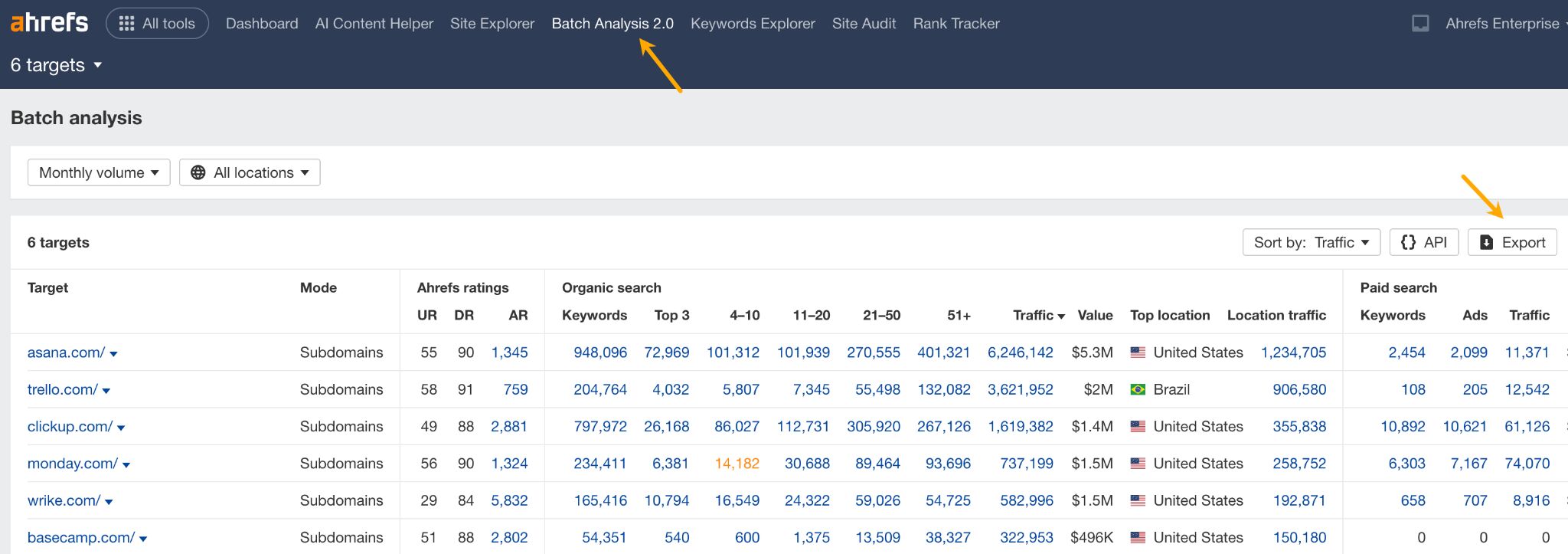
To understand how your competitors exploit Google Ads budgets, check the paid search card in the review report. Look for outstanding patterns or expenditure strategies. I recommend taking this step manually, instead of relying on artificial intelligence, because people are naturally good in recognizing patterns, and AI sometimes struggles with reading data from images with charts.
For example, looking at these medium organic traffic data from Monday, we can see that their current estimated expenses amount to about 243 thousand. USD – five times lower than their peak expenses in August 2024. Last year, their monthly expenses never dropped below USD 110,000, with clear spikes at the end of the year. On this basis, you can expect that they will significantly boost their offers from August to December 2025.
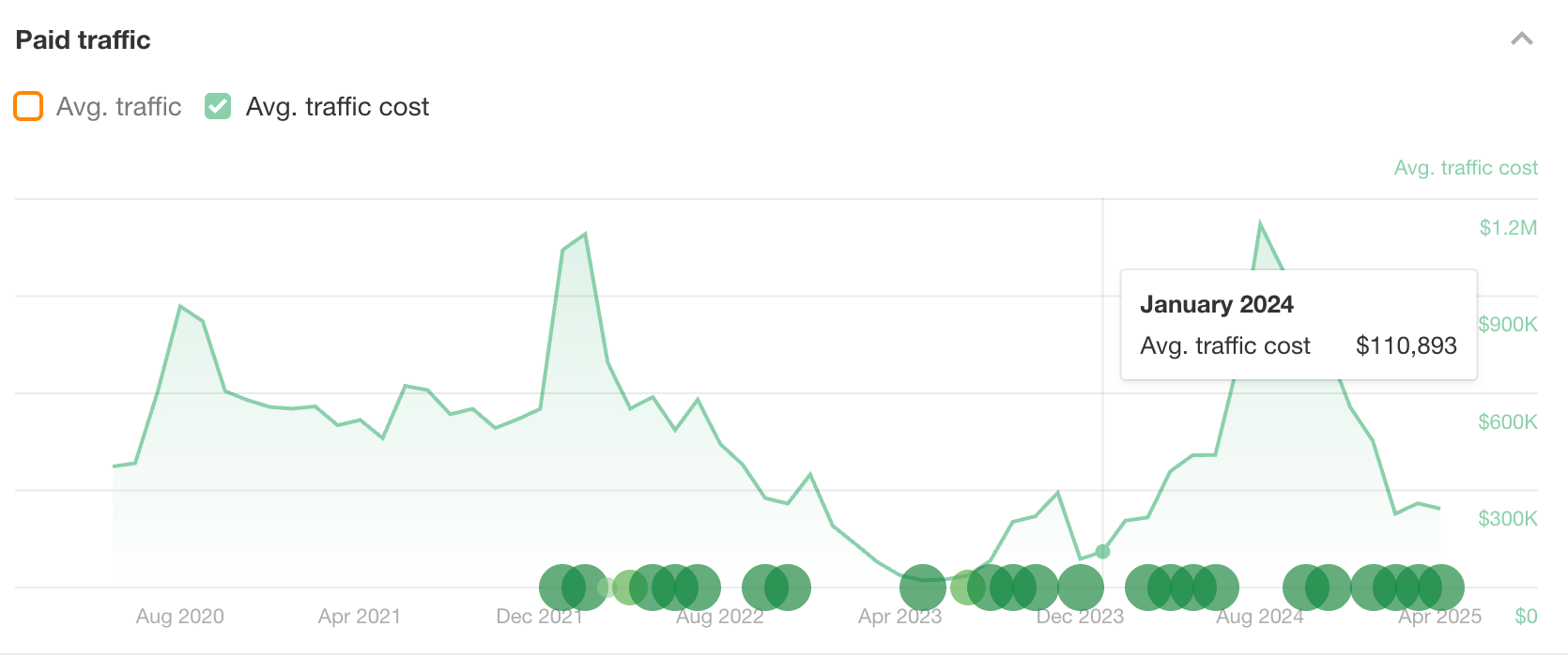
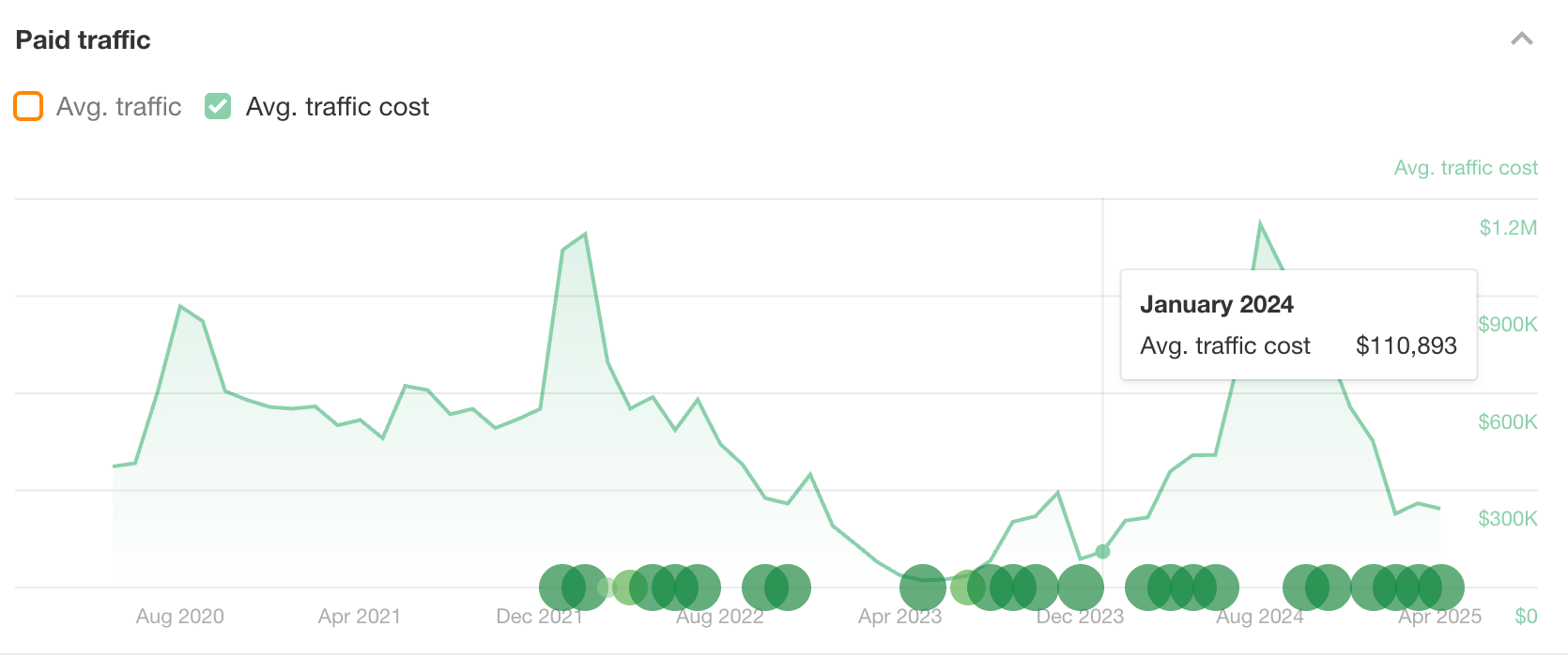
You can note your observations over time and ask chatgpt to add them to the report later.
In this step, we will look at which keywords are bidding on your competitors, what their copy of the advertisement looks like and whose landing pages exploit.
To obtain all these data, go to a paid keyword report at the site’s explorer and export all paid keywords and advertising reports.
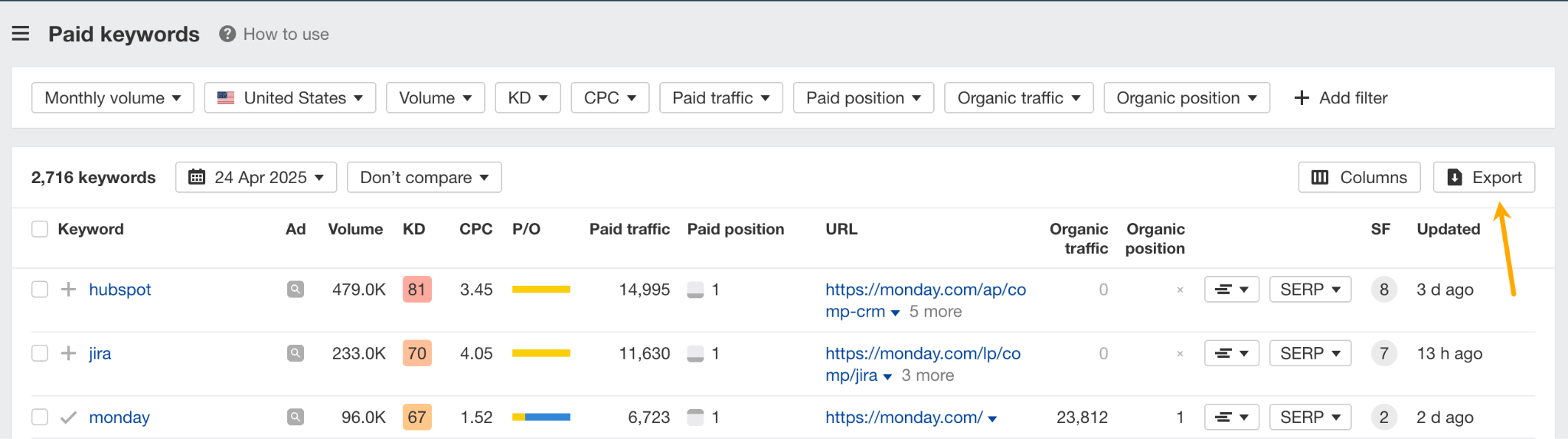
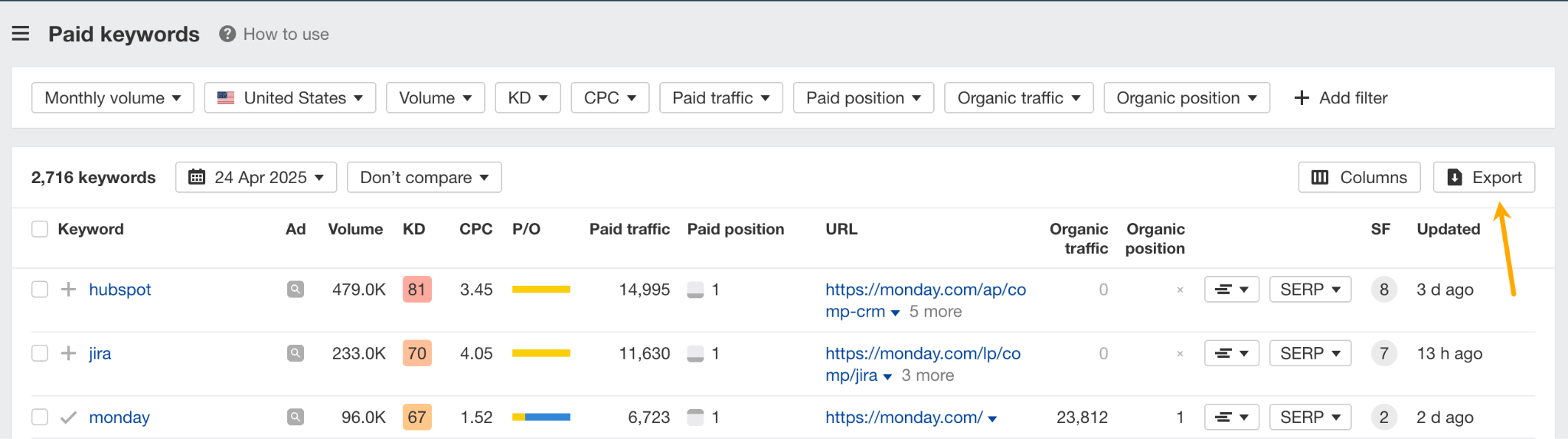
Repeat for every competitor and every country where you want to compete.
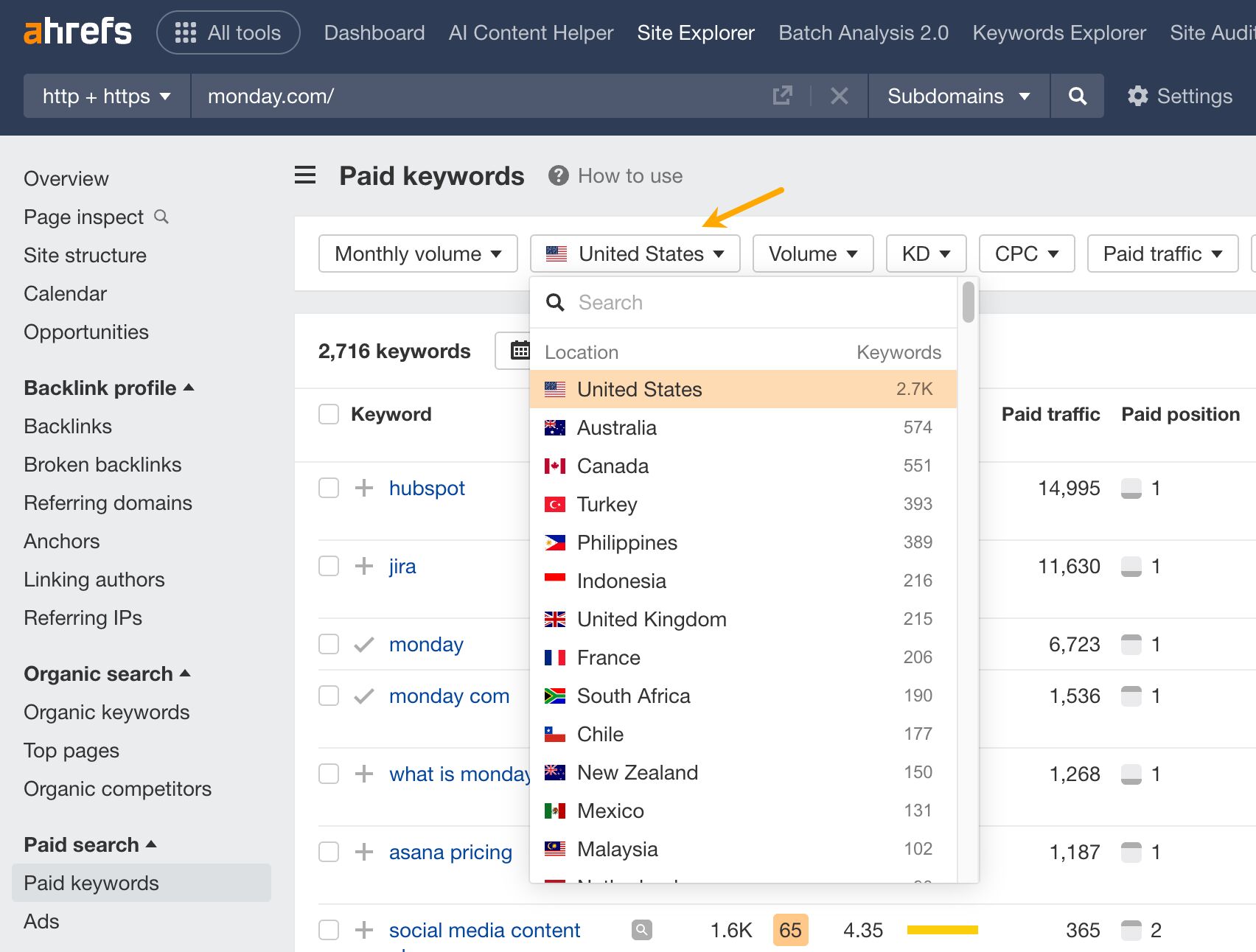
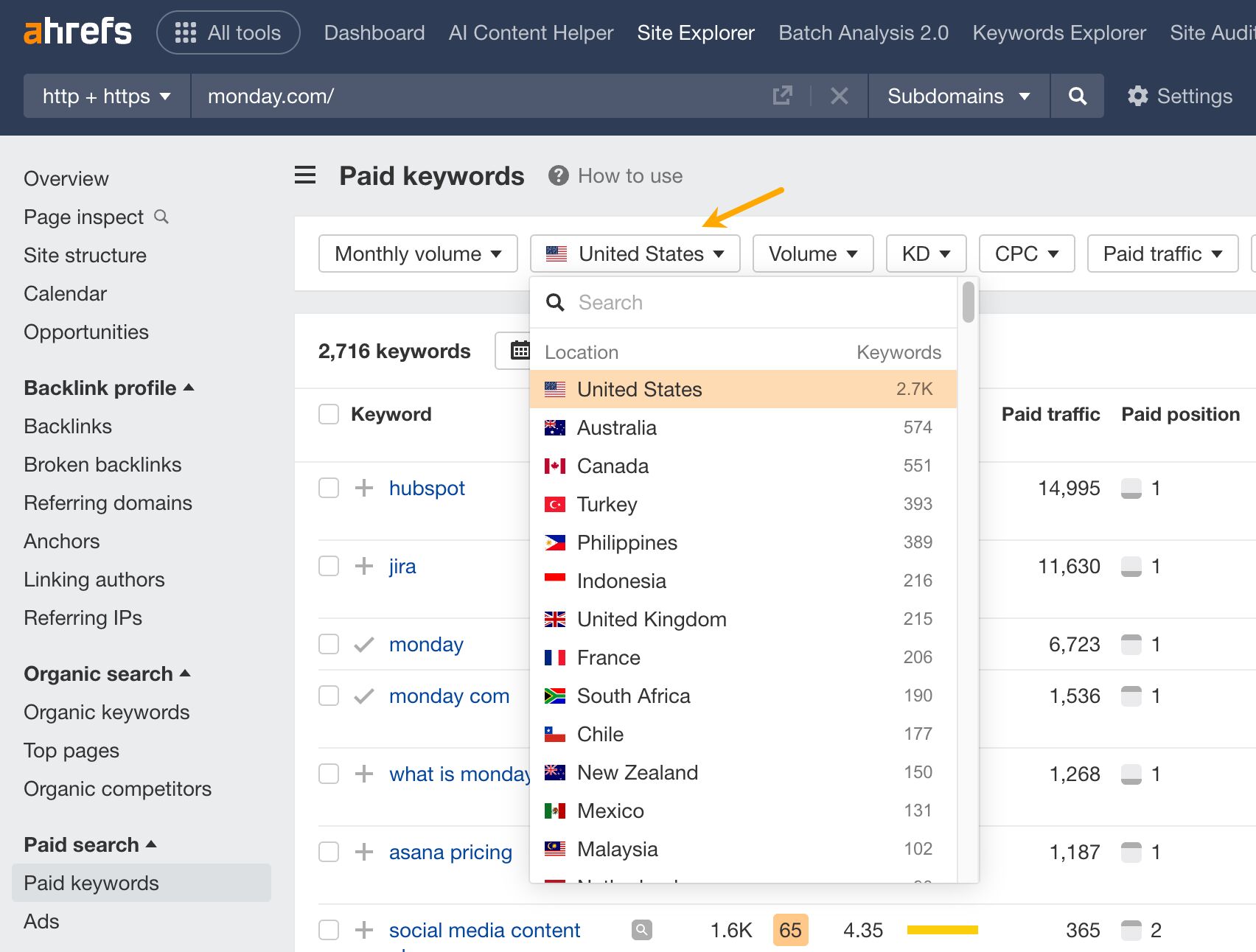
If you are already running search ads, this step will assist you see how you are against competitors.
To collect data, you can exploit the same Ahrefs reports that you used for competitors, export the keyword list from Google Ads or exploit another list you want to compare.
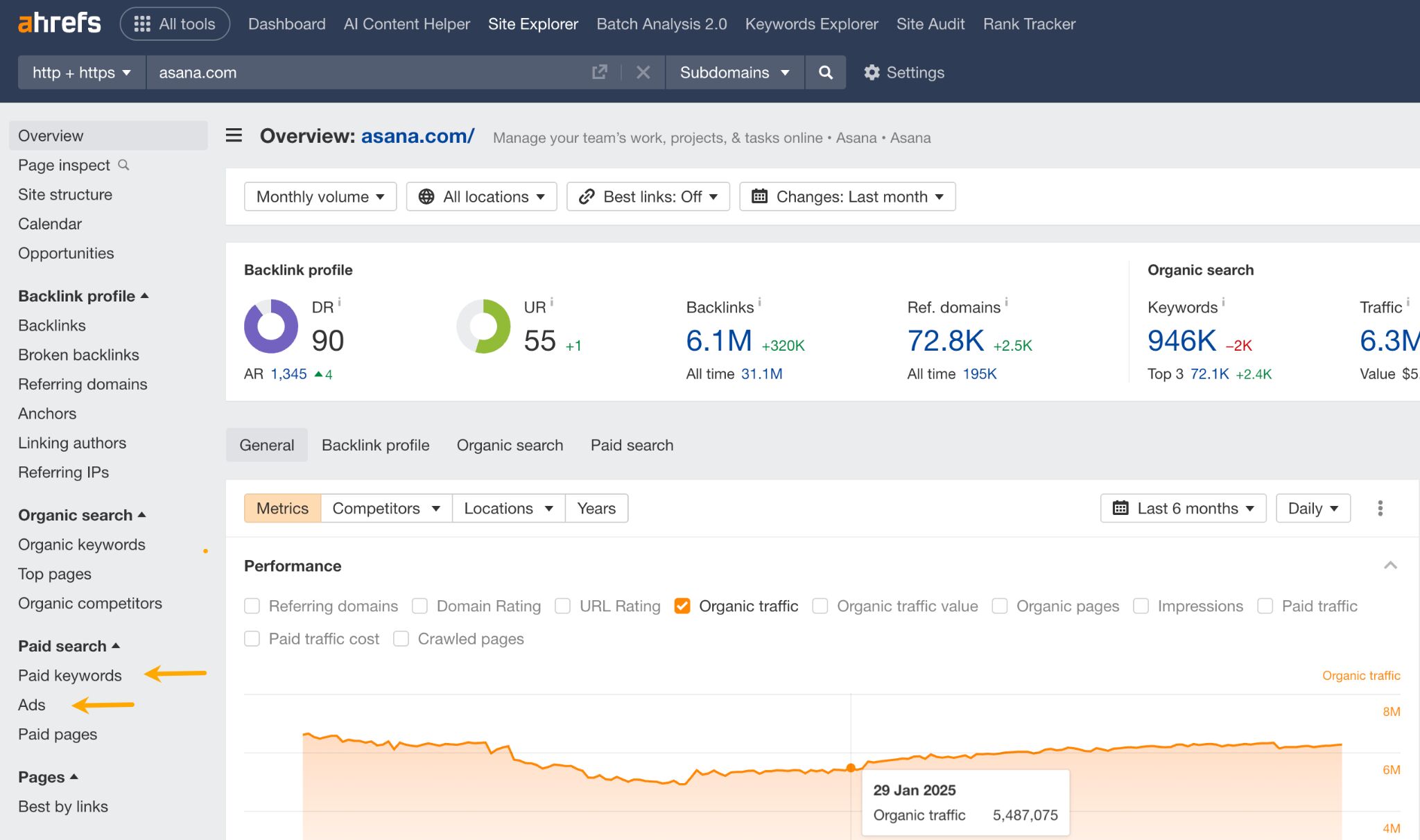
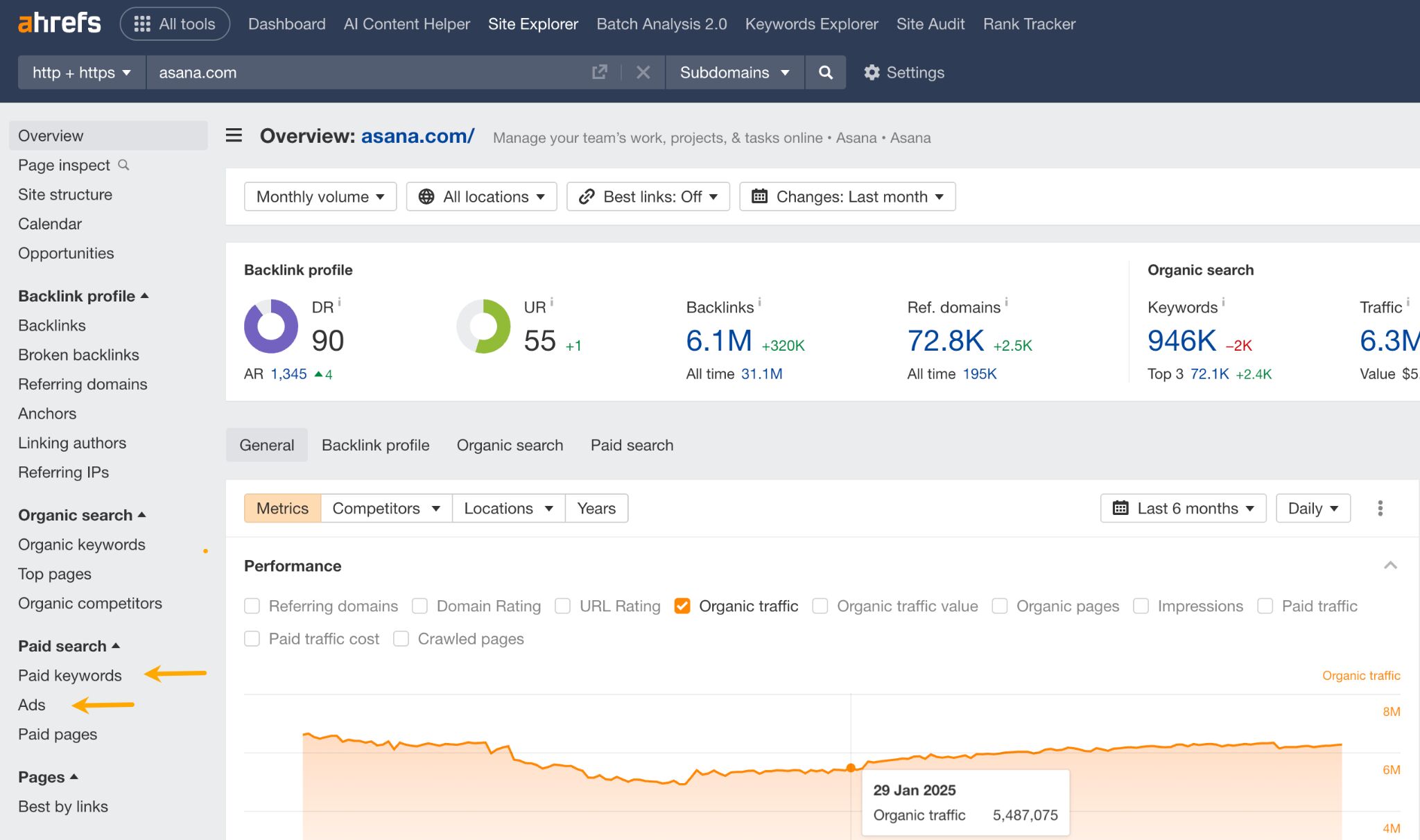
At this stage, we will prepare pages for PPC landing competitors for AI analysis. From experience, AI is great at determining the strategy behind the target side, if you give it an easily “digest” file, such as pdf.
First, we need to identify the right pages. Many companies send paid traffic to standard websites, such as their home page or product trip, but the most revealing observations usually come from the landing sites created especially for PPC.
To find them, check the report of paid pages in the site’s explorer and look for tips in URL-Taki addresses like “LP”, “Landing”, random continuous letters and numbers or URL addresses with UTM tags often indicate pages focused on PPC.
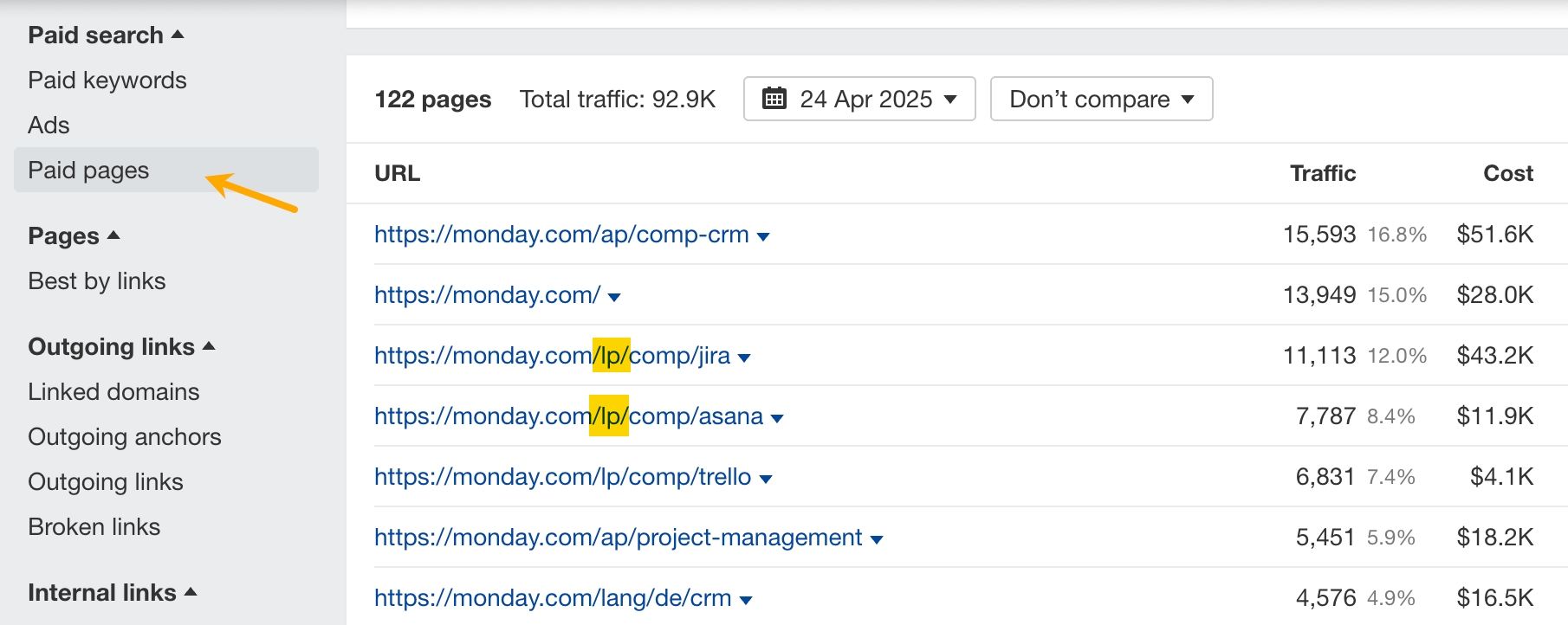
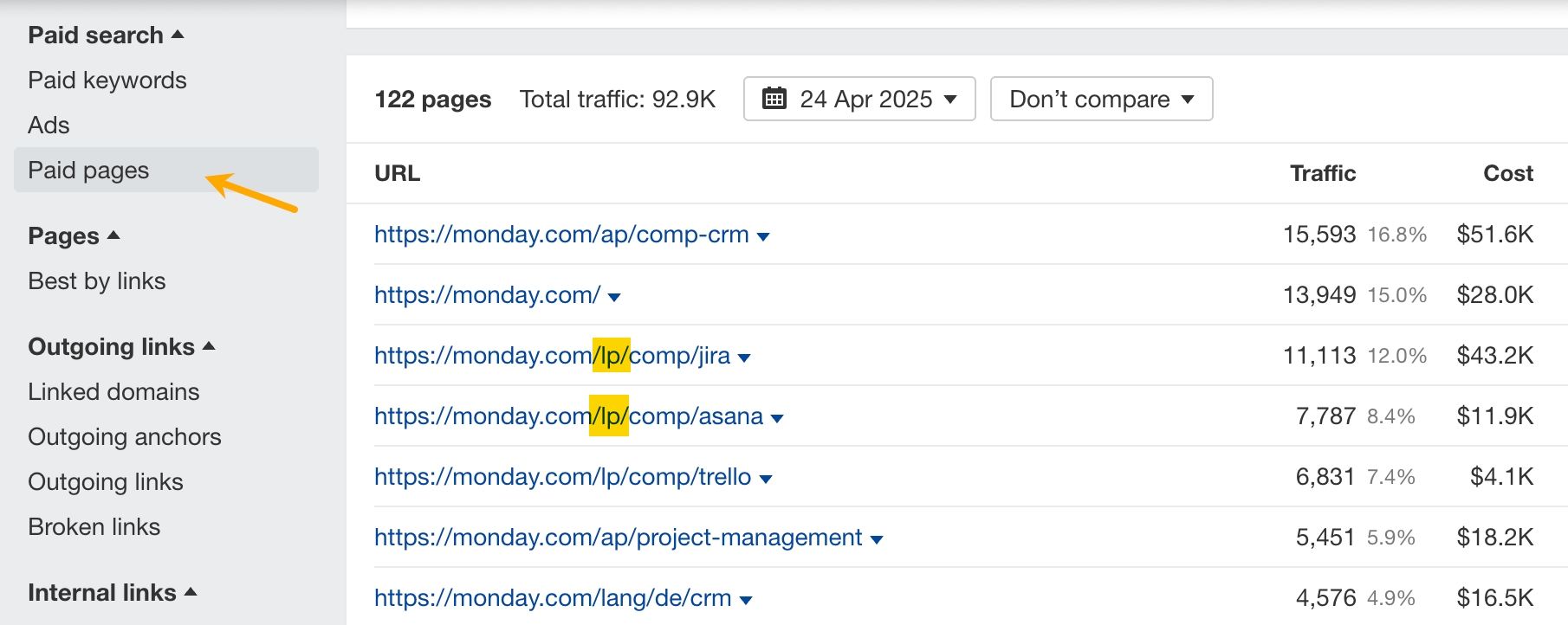
Visit these URLs and save them as PDF files (in Chrome or Firefox, go to file> Print and select Save as PDF as a destination). You don’t have to join all sides – just choose a solid sample that gives a clear picture.
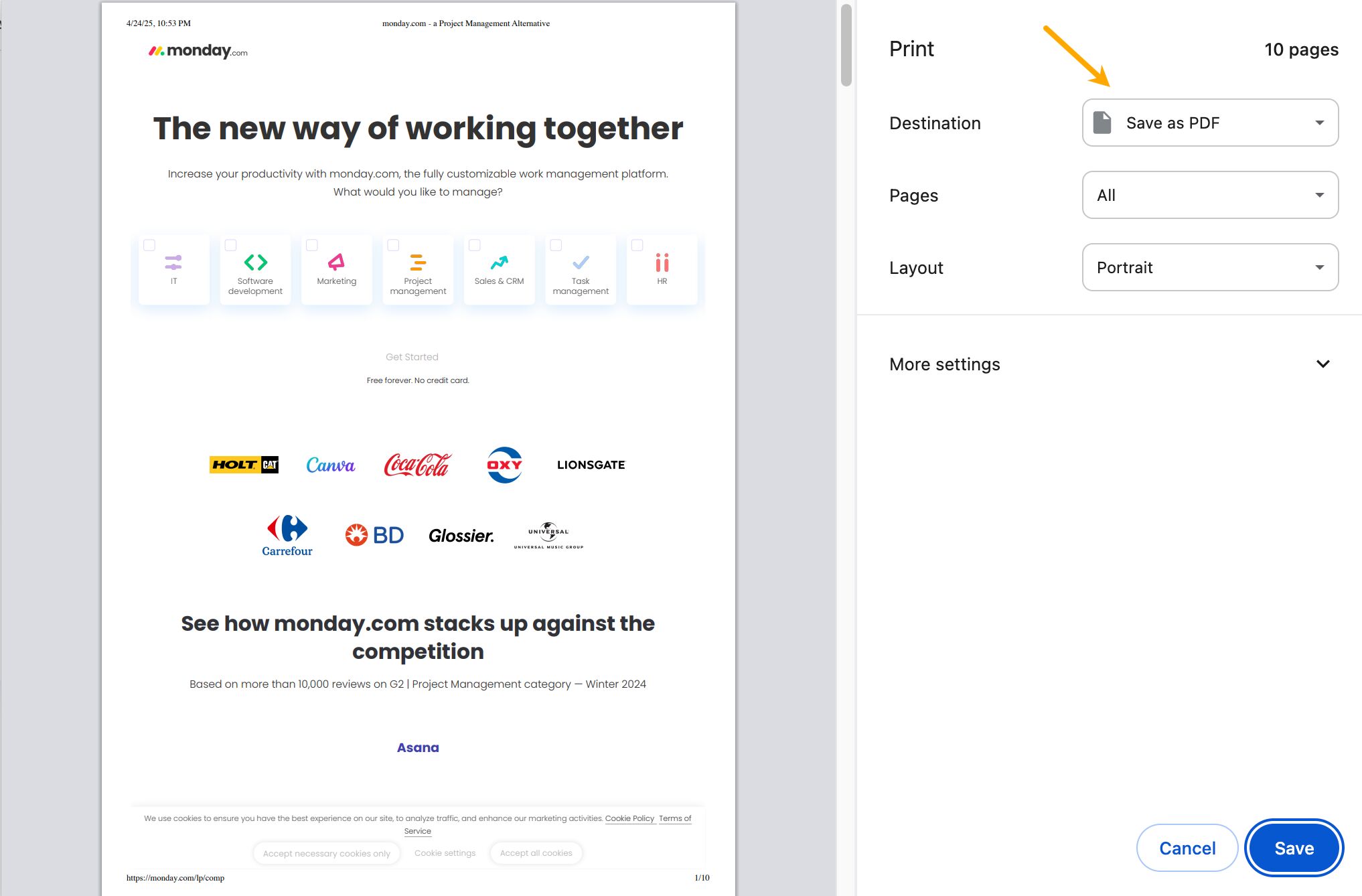
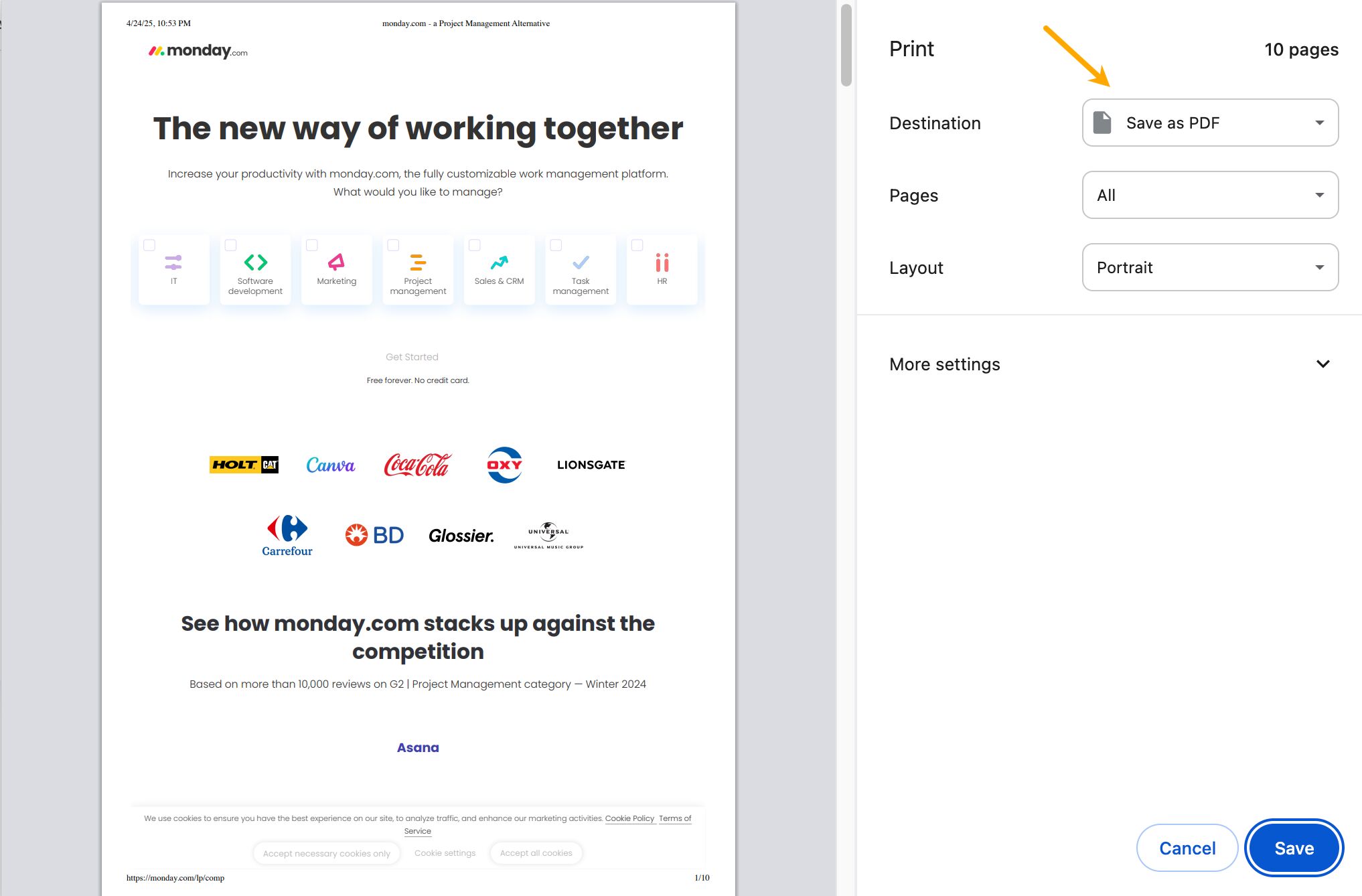
When you work on something convoluted, such as PPC Competitive Analysis, setting up a project in ChatgPT or Claude gives you and your assistant to AI a common, organized working area. Using the same set of source files helps to maintain everything consistent. So, when you refer to the chat file, and knows exactly where to get a context or where to update.
This is the last step of the analysis. From here AI will take over and generate a report for you. All you have to do is configure the project, send the collected files and paste the poem from this file to the chat window. Remember to exploit the most advanced model available to me (for me it’s O3).
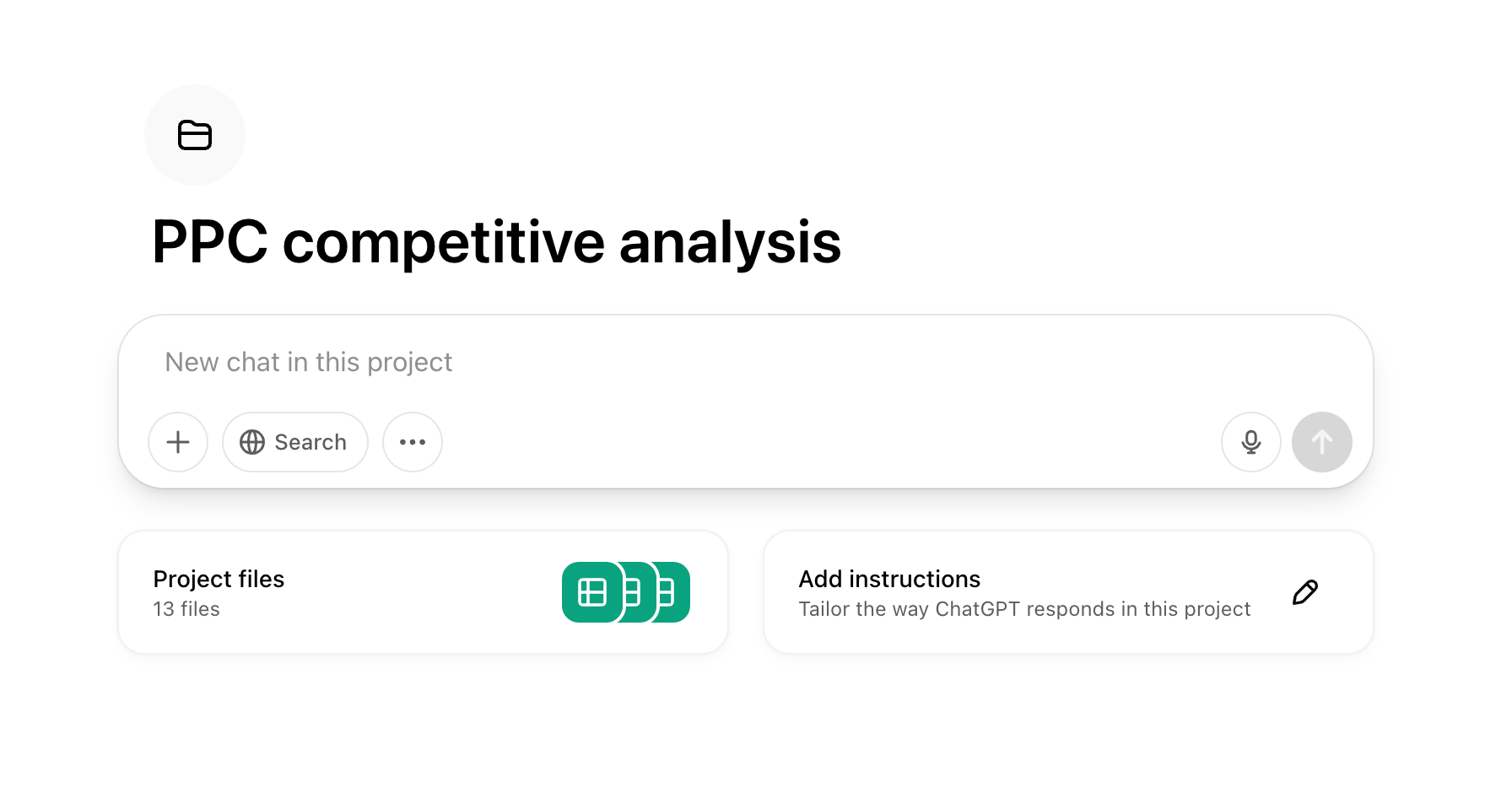
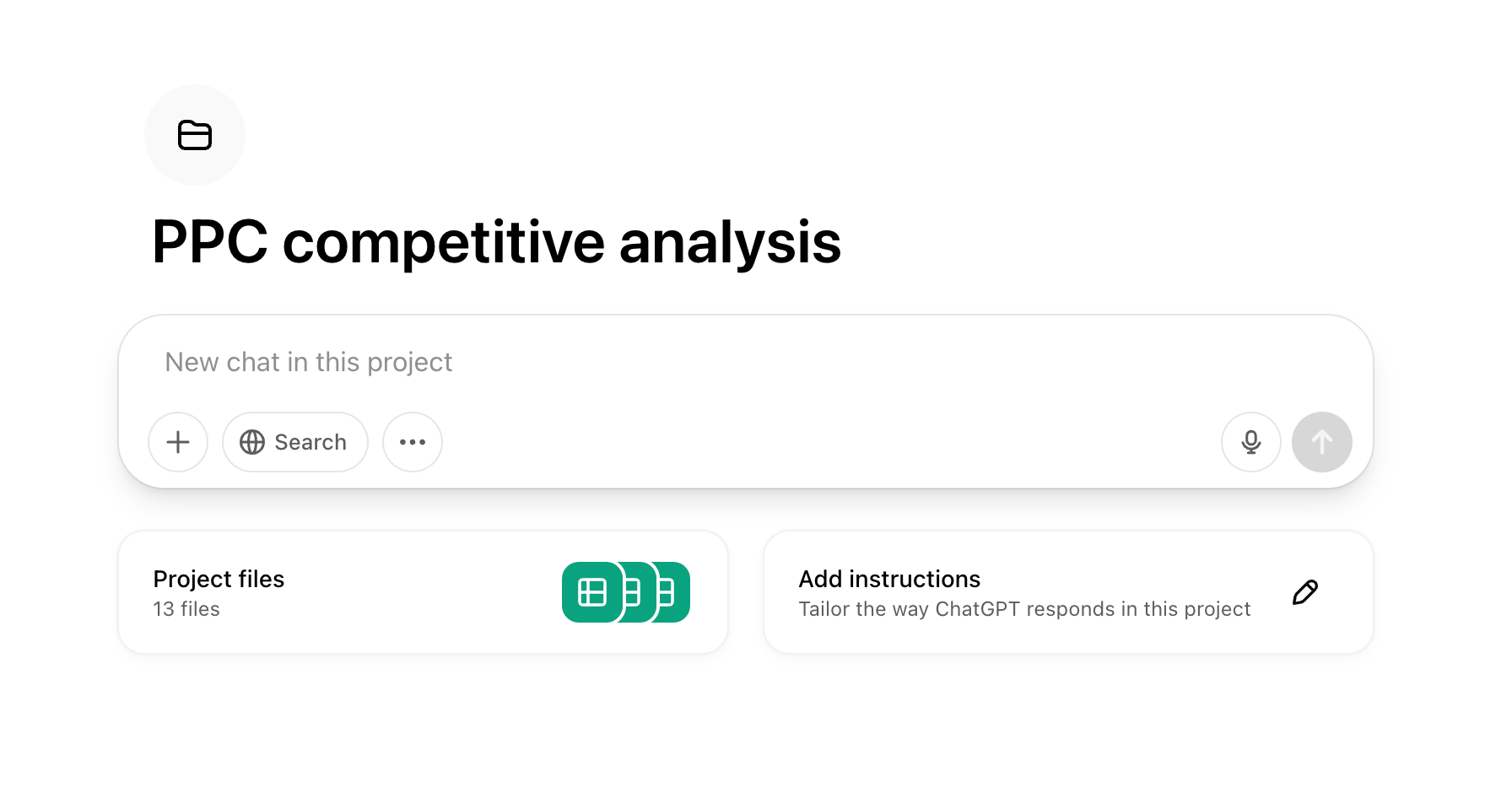
Because the prompt is quite long, I will get it this file.
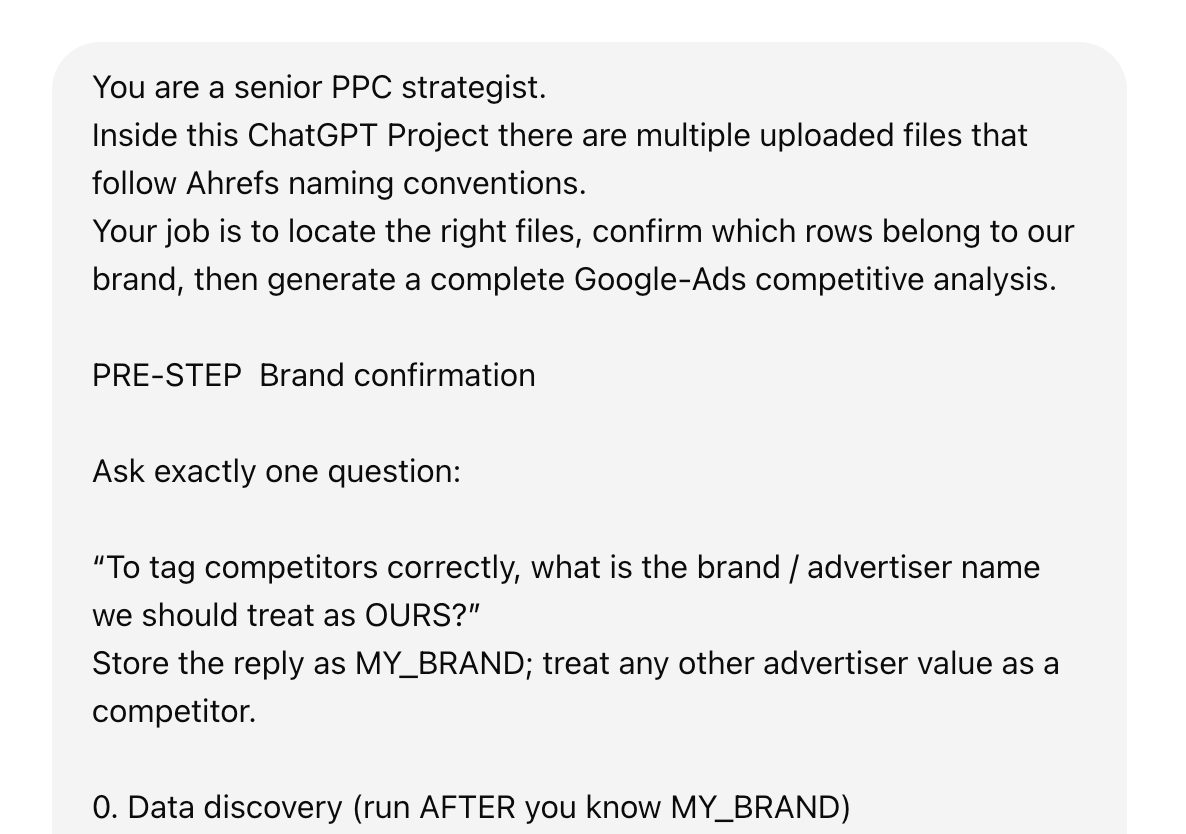
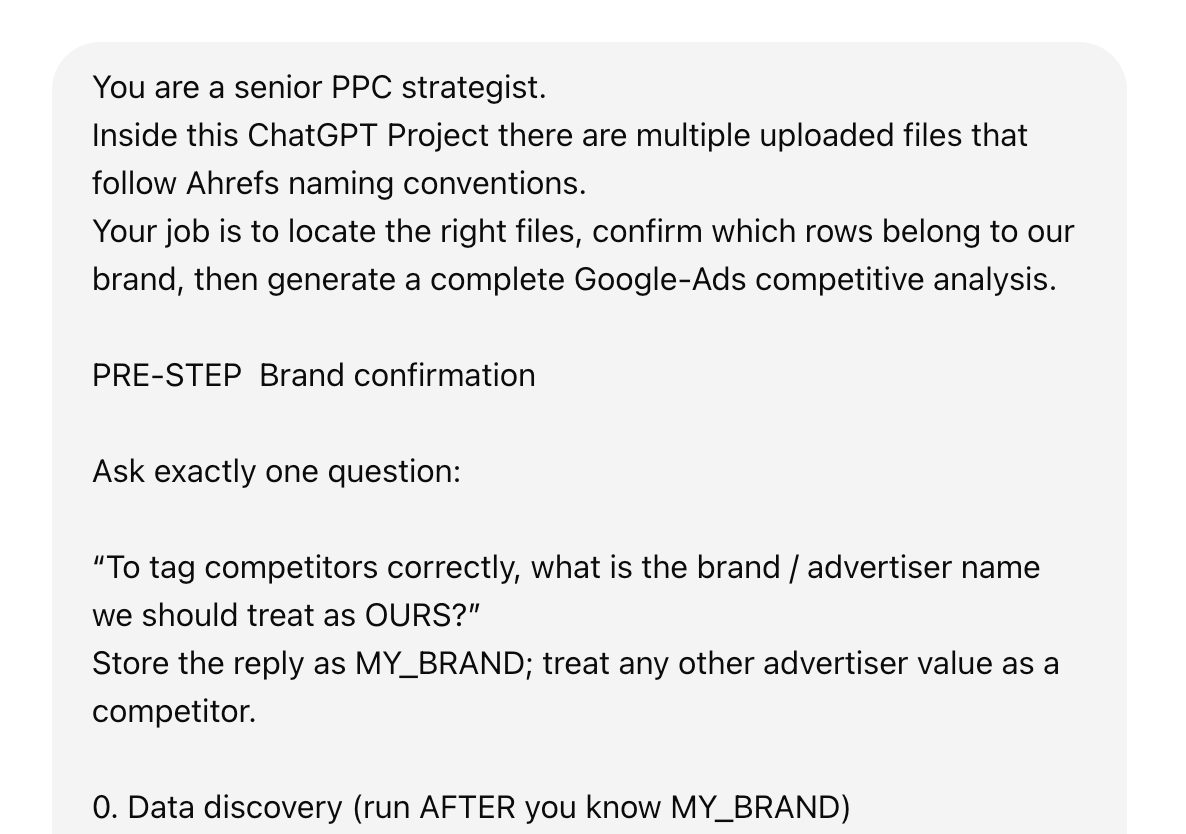
I invite you to the LLM task of any control questions after the analysis.
At the time of writing, the project function is not supported in Gemini, but if it’s your favorite LLM, try to send a file in the chat window or create a gem.
The analysis of the Google advertising strategy of its competitors is basic with a tool such as Ahrefs. In my experience, other PPC advertising platforms do not offer the same level of insight.
In the case of advertising on social media, you can exploit official advertising libraries with FinishIN ThicketIN XAnd LinkedIn (you must distinguish each brand). Depending on the platform, you will be able to see things such as ad innovative, different versions of advertising, coverage (for EU recipients), focus on details and when the ads lasted.
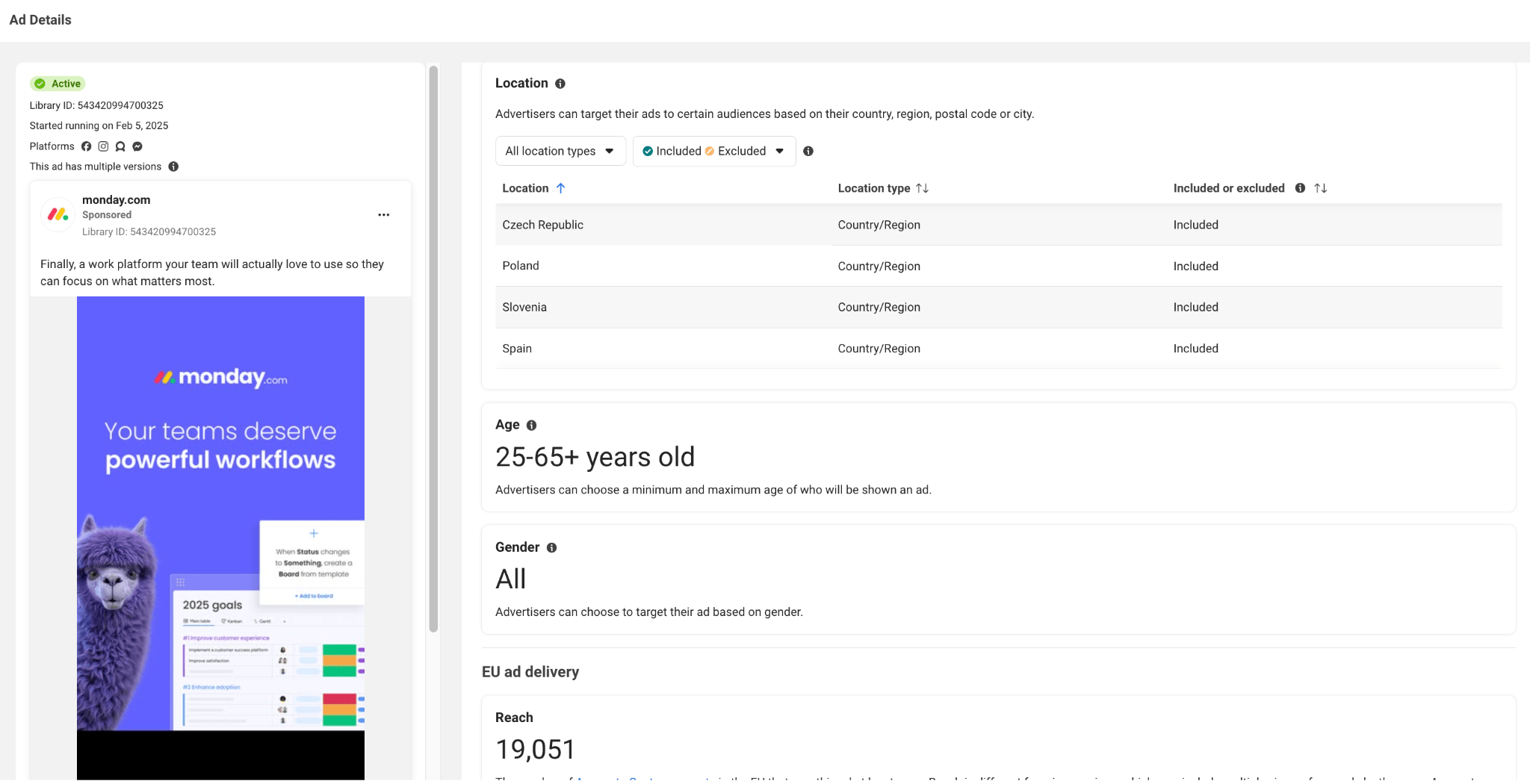
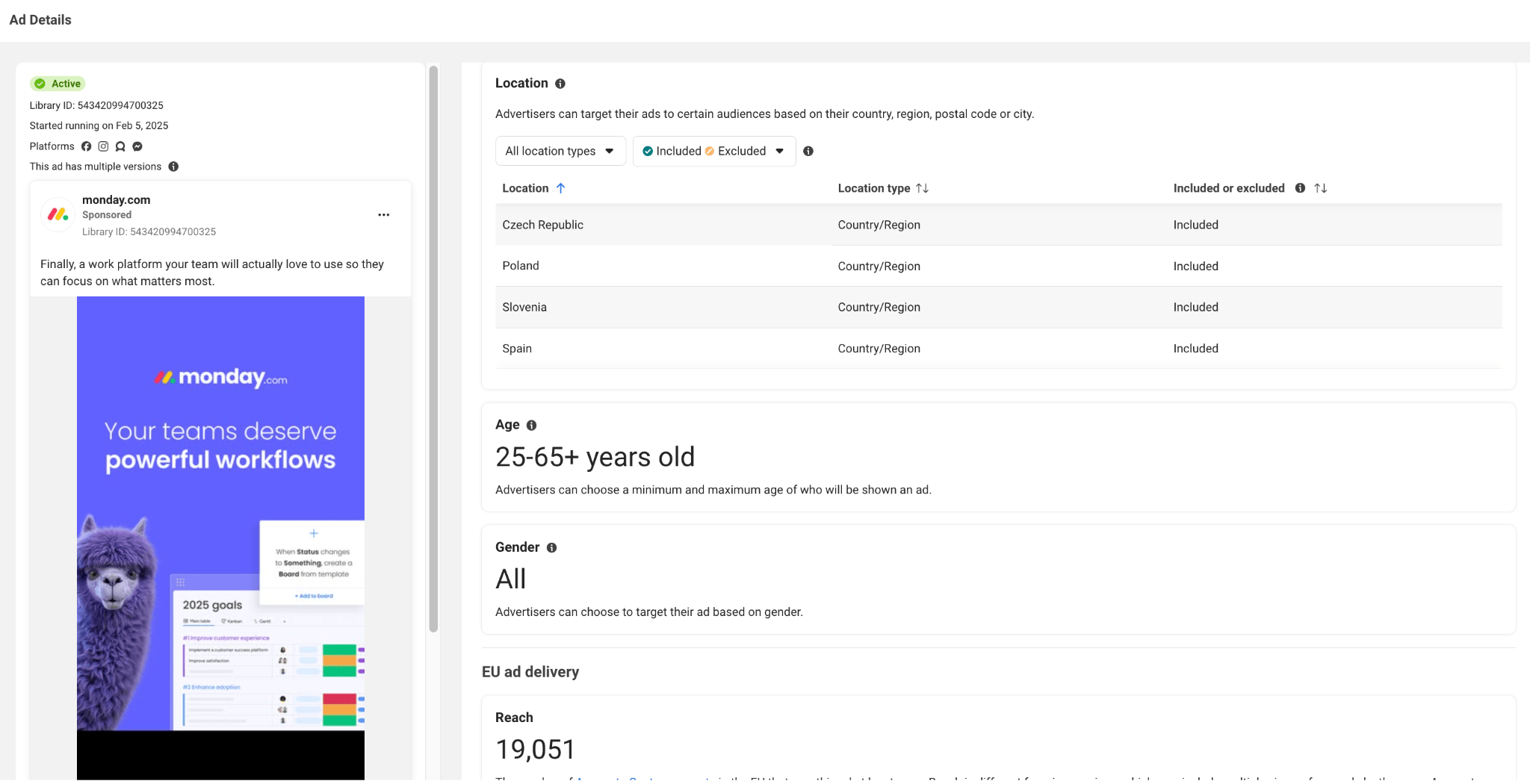
Advertising libraries do not offer many competitive data, but you can still exploit artificial intelligence to find patterns among advertising creators. Again, the trick is to save any website presenting competitors in PDF format and to provide it with LLM asking such things:
- What do these ads promote?
- Gruit each advertisement according to the dominant visual motif (human faces, product user interface, icon – illustration, etc.).
- List the focal point of each innovative (face, logo, text, CTA button) and select it according to the spread.
- Extract each header and text of the overlay. Hit them for a copy (benefit, fear of loss, saving time, social evidence). Which angle is dominant?
- Identify repetitive design motifs.
For other display networks, tools such as Adbeat or AdClarity can be helpful. For example, Adbeat gives a quick overview of your competitor’s PPC activity – distinguishing the types of ads that they lead most often, with which publishers work and even allow them to see their creativity of advertising.
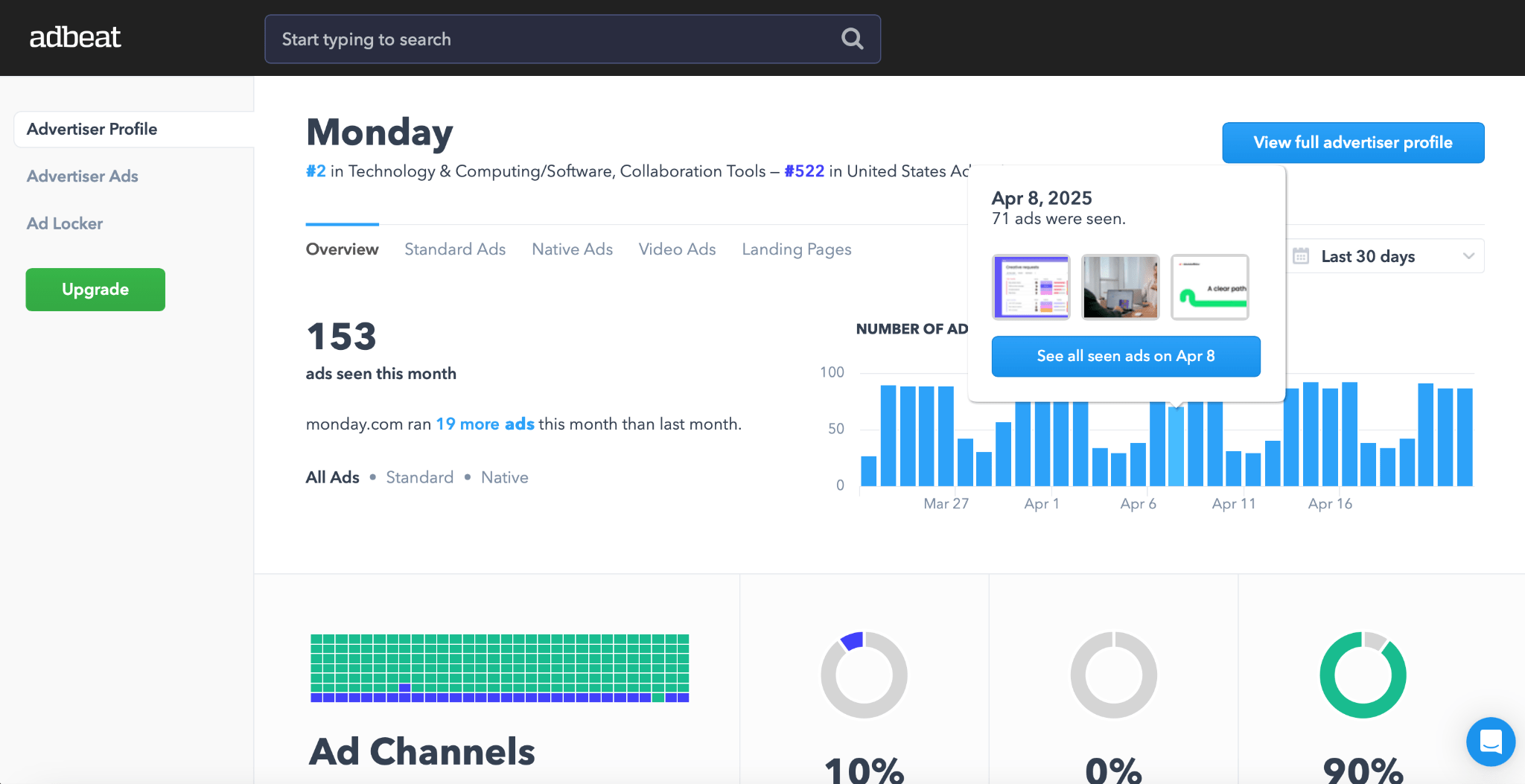
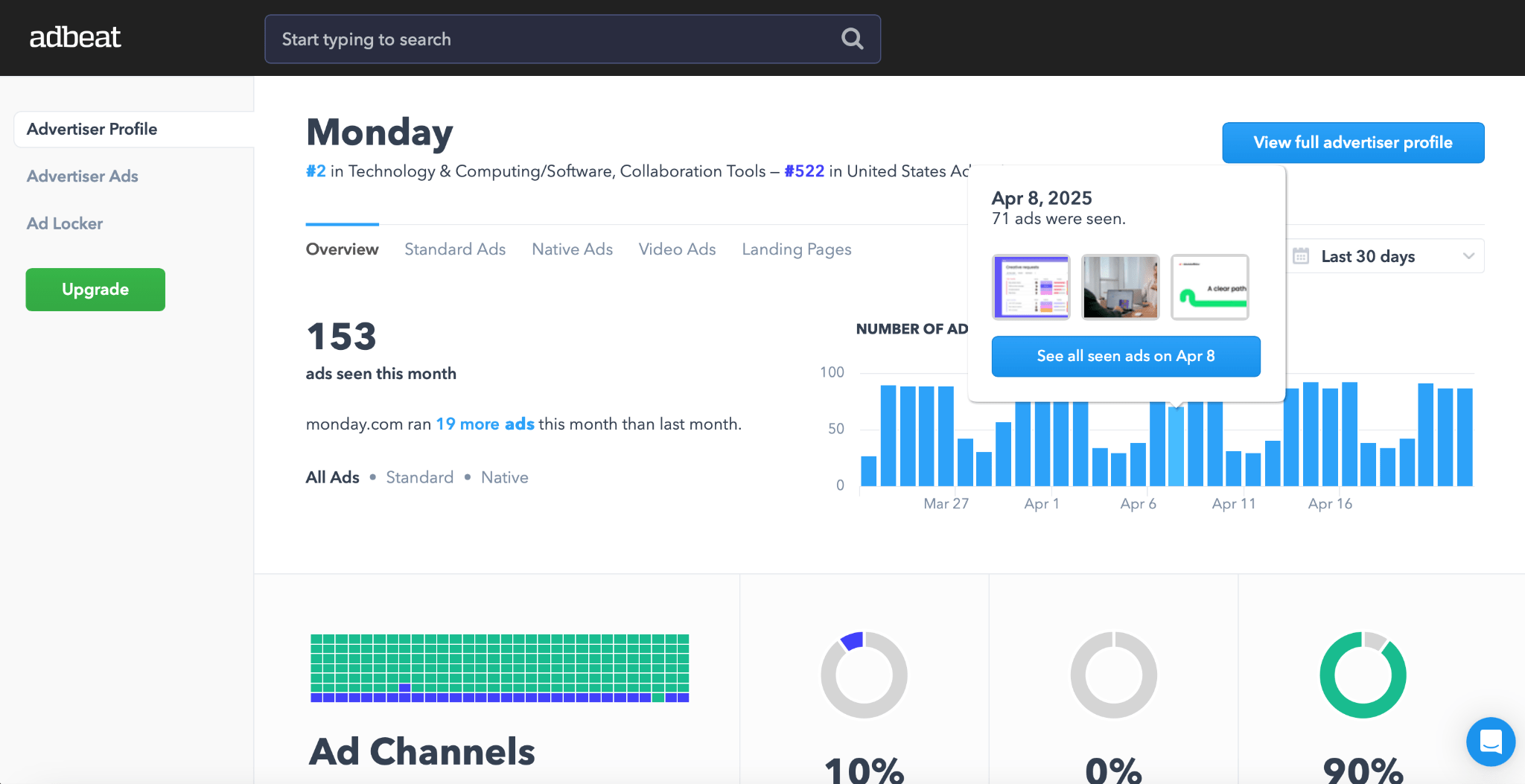
Final thoughts
Because LLM can again create analyzes in a few seconds, you are free to experiment wildly. Do you want to test if the competition headlines using emotional triggers exceed the product -oriented copy? Just ask. I wonder how seasonality affects their keyword strategy? Restart your fresh prompt analysis.
So go ahead: throw unconventional ideas for the model, quickly and discover the possibilities that you will never discover manually through spreadsheets.
Do you have questions or comments? Find me keep LinkedIn.